Businesses of all sizes and in all sectors are increasingly using data to guide their decision-making in today’s data-driven environment. Data management has so become a significant element for many enterprises and activities. The idea of a “data supply chain” has thus emerged as a crucial framework for managing and utilizing data efficiently.
“Data supply chain” refers to the end-to-end flow of data, from its initial collection and processing to its final utilization and analysis. Similar to a traditional supply chain, a data supply chain comprises various processes, technologies, and stakeholders collaborating to ensure the timely and accurate delivery of data to the intended destination in the correct format.
Almost all successful data supply chain efforts share a common characteristic: a strong emphasis on data quality, regardless of the wide range of methodologies and tactics available.
Data quality refers to the precision, completeness, diligence, and credibility of data. Without high-quality data, it is difficult to gain meaningful insights or make informed decisions, and organizations may struggle to fully leverage the potential of their data.
This article will explore the importance of data quality in the context of data supply chains and examine several crucial tactics and best practices that businesses can implement to ensure they build their data supply chains on a strong foundation of high-quality data.
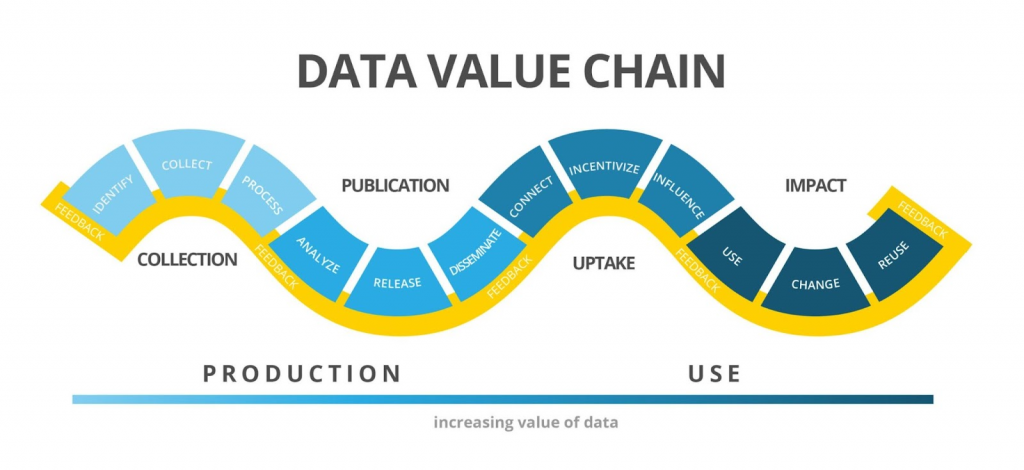
Image – CC BY 4.0 International license-free use with Attribution
Why Data Quality Matters in Data Supply Chains
Data supply chains are the backbone of modern businesses. They involve the collection, storage, processing, and analysis of vast amounts of data from a variety of sources, including customers, vendors, partners, and internal operations. The success of these data supply chains depends on the quality of the data that flows through them.
Data quality refers to the degree to which data is accurate, complete, consistent, timely, and relevant for its intended use. Poor data quality can lead to incorrect insights, flawed decisions, and missed opportunities. Additionally, it can damage a business’s reputation, lose consumer confidence, and result in legal and regulatory problems.
In the context of data supply chains, data quality matters for several reasons:
1. Accurate insights for the Data supply chain:
Data supply chains are designed to derive insights from data. However, if the data is of poor quality, the insights generated will be inaccurate, incomplete, or misleading. This can result in poor decisions and lost opportunities.
2. Efficient operations:
Operations might become inefficient as a result of bad data quality. Inaccurate client data, for instance, might lead to inaccurate billing, delayed shipping, and subpar customer service. Costs might go up, and consumer relationships can suffer.
3. Compliance:
Many industries are subject to regulatory requirements that require the accuracy and completeness of data. Poor data quality can lead to compliance issues, fines, and legal liabilities.
4. Reputation:
Poor data quality can harm a company’s reputation, especially in industries where data privacy and security are paramount. Customers and stakeholders expect companies to handle their data with care and respect, and poor data quality can erode trust and damage a company’s brand.
To ensure data quality in data supply chains, companies need to adopt a holistic approach that includes data governance, data management, and data integration strategies. This involves establishing data quality standards, implementing data quality checks, and investing in data quality tools and technologies.
In summary, data quality is critical to the success of data supply chains. Companies that prioritize data quality are more likely to derive accurate insights, improve decision-making, and build trust with their customers and stakeholders. In contrast, companies that neglect data quality run the risk of making poor decisions, eroding customer trust, and harming their reputation.
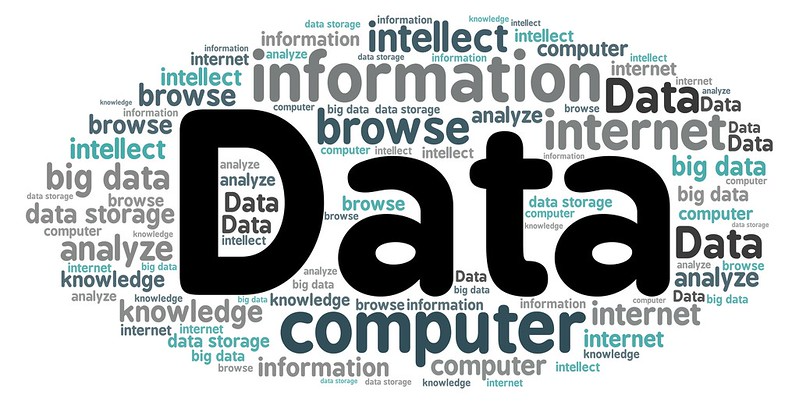
Photo by flickr
Key Strategies for Ensuring Data Quality in Data Supply Chains
Data quality is a critical component of any data supply chain. Without high-quality data, downstream analytics and decision-making may be compromised, leading to poor business outcomes. Therefore, organizations must prioritize data quality when designing and managing their data supply chains. In this article, we’ll explore some key strategies for ensuring data quality in data supply chains.
1. Define data quality standards for the data supply chain:
Data quality standards should be defined and documented to ensure that all stakeholders understand what constitutes high-quality data. These standards should outline specific requirements for data accuracy, completeness, timeliness, consistency, and validity. By establishing clear standards, organizations can minimize misunderstandings and ensure that data quality expectations are consistently met.
2. Establish data governance policies for the data supply chain :
Data governance policies help ensure that data quality standards are followed and that the data supply chain is properly managed. These policies should include guidelines for data ownership, data stewardship, and data lifecycle management. By establishing clear policies and procedures, organizations can help ensure that data is properly managed throughout its lifecycle, from creation to disposal.
3. Conduct data profiling :
Data profiling involves analyzing data to understand its characteristics and identify potential quality issues. This process can help organizations identify data anomalies, such as missing values, incorrect formats, or inconsistent data, that can affect data quality. By conducting data profiling, organizations can take proactive steps to address data quality issues and improve the overall quality of their data supply chain.
4. Implement data validation :
Data validation involves checking data against predefined rules to ensure that it meets specific quality standards. This process can help organizations identify data quality issues in real time and take corrective action. Data validation can be automated, which can help reduce errors and improve efficiency. By implementing data validation, organizations can ensure that only high-quality data is used in downstream analytics and decision-making.
5. Establish data quality metrics :
Data quality metrics provide a way to measure and monitor data quality over time. These metrics should align with the data quality standards established by the organization and be tracked regularly to ensure that data quality goals are being met. By establishing data quality metrics, organizations can identify areas for improvement and take corrective action to ensure that data quality remains high.
6. Implement data cleansing :
Data cleansing involves identifying and correcting errors in data. This process can help organizations improve the accuracy and completeness of their data and reduce the risk of downstream issues. Data cleansing can be automated, which can help reduce errors and improve efficiency. By implementing data cleansing, organizations can improve the overall quality of their data supply chain and ensure that downstream analytics and decision-making are based on high-quality data.
7. Implement data integration:
Data integration involves combining data from multiple sources into a unified view. This process can help organizations improve the completeness and consistency of their data and reduce the risk of downstream issues. Data integration can be automated, which can help reduce errors and improve efficiency. By implementing data integration, organizations can improve the overall quality of their data supply chain and ensure that downstream analytics and decision-making are based on a unified and complete view of the data.
8. Conduct data auditing :
Data auditing involves reviewing data to ensure that it meets specific quality standards. This process can help organizations identify data quality issues and take corrective action. Data auditing can be automated, which can help reduce errors and improve efficiency. By conducting data auditing, organizations can ensure that data quality remains high and that downstream analytics and decision-making are based on high-quality data.
In conclusion, data quality is critical for the success of any data supply chain. By defining data quality standards, establishing data governance policies, conducting data profiling, implementing data validation, establishing data quality metrics, implementing data cleansing, implementing data integration, and conducting data auditing, organizations can ensure that their data supply chains are of high quality and that downstream analytics and decision-making are based on high-quality data. By prioritizing data quality,
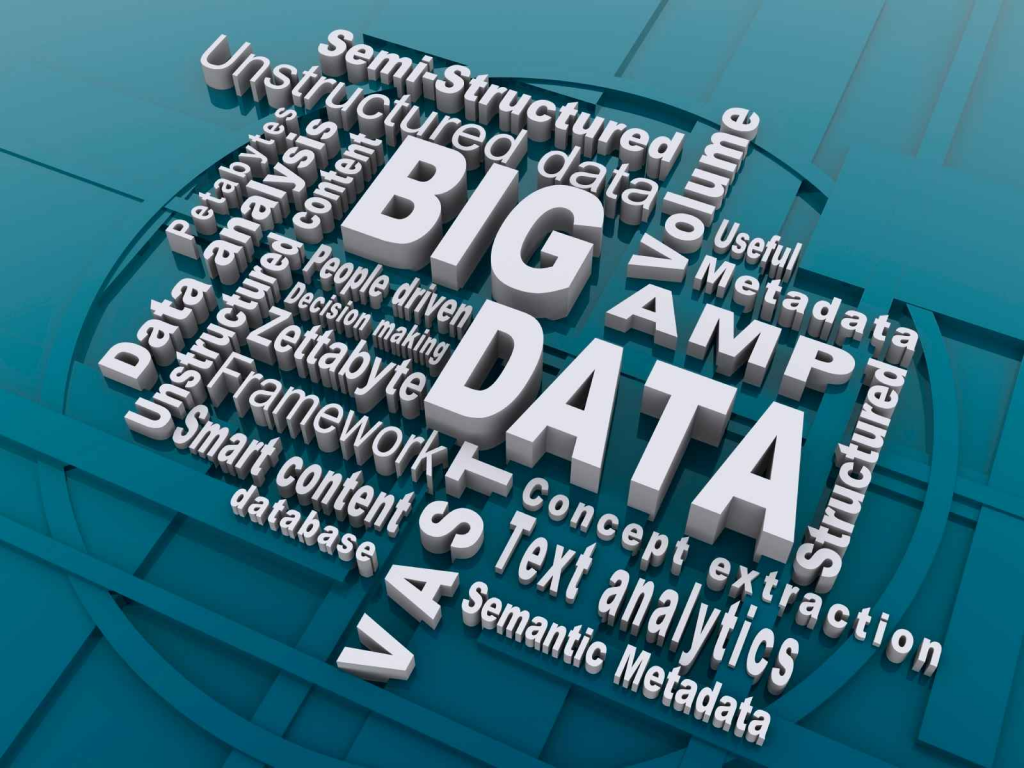
Photo by Learntek, CC0, via Wikimedia Commons
In conclusion
The one thing that all data supply chain success stories have in common is a focus on data quality. Ensuring data quality requires a holistic approach that encompasses data governance, data management, and data integration strategies. Companies that prioritize data quality in their data supply chains are more likely to derive actionable insights, improve decision-making, and gain a competitive edge in the market. They are also more likely to build trust with their customers and stakeholders, who increasingly demand transparency and accountability in the use of data.
Data quality is not a destination that can be reached in a single step, but rather a process that involves constant monitoring, assessment, and improvement. Collaboration with internal and external partners and vendors, as well as stakeholders from various departments and stakeholders, is necessary. The need to invest in data quality in the data supply chain has become imperative due to the growing significance of data in determining corporate performance. Businesses will be better positioned to thrive in the data-driven economy of the future if they recognize this and take the necessary steps to improve the quality of their data.