Datafication, the process of converting human activity into digital data, has become increasingly prevalent across many industries in recent years. In healthcare, datafication has the potential to revolutionize patient care by providing clinicians with valuable insights into patient health. Enabling more accurate diagnoses, and improving treatment outcomes. This article will explore the role of data analytics in healthcare. With a focus on electronic health records (EHRs), predictive analytics, and the ethical implications of datafication. We will also examine the potential for datafication to improve patient outcomes and transform the healthcare industry.
The Role of Data Analytics in Healthcare
Data analytics is the process of analyzing data sets to draw insights and make informed decisions. In healthcare, practitioners can use data analytics to improve patient outcomes by identifying patterns and trends in patient health data. This can help clinicians make more accurate diagnoses, develop more effective treatment plans, and monitor patient progress over time.
One example of data analytics in healthcare is machine learning. A type of artificial intelligence that allows computer systems to learn from data and improve their performance over time. Machine learning algorithms can analyze large data sets, such as medical images or EHRs. To identify patterns that may not be apparent to human clinicians. This can help identify potential health risks or diagnose diseases at an early stage, improving patient outcomes.
Another example of data analytics in healthcare is predictive analytics. Which involves using statistical models to predict future events or outcomes. Healthcare professionals can use predictive analytics to predict patient outcomes. Such as the likelihood of readmission, patient deterioration, or mortality. This can help clinicians identify high-risk patients and intervene before complications occur, improving patient outcomes and reducing costs.
Healthcare Data Sources
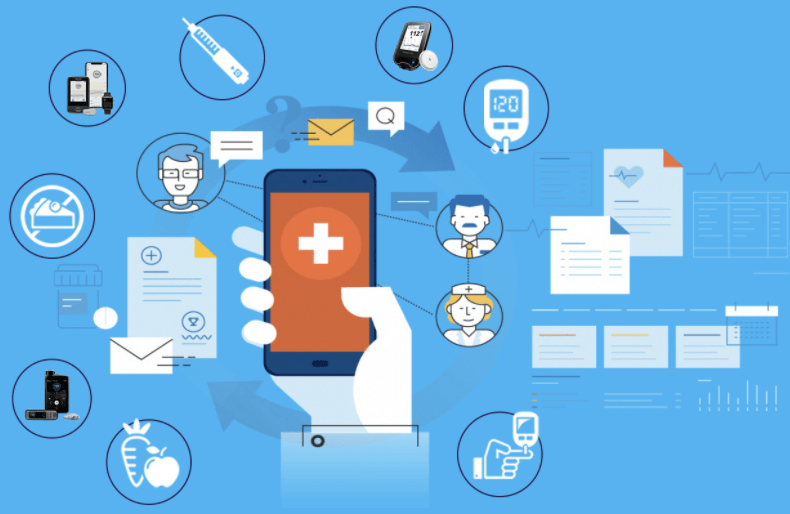
Photo by Triera Zekaj on Wikimedia Commons
- Electronic health records (EHRs): EHRs are digital records of a patient’s medical history, including diagnoses, medications, lab results, and imaging data. EHRs are the primary source of patient data in healthcare. They can be used to improve clinical decision-making, reduce errors, and improve patient safety.
- Claims data: Claims data refers to data from insurance claims, including information on diagnoses, treatments, and costs. Claims data can be used to identify trends in healthcare utilization and costs. It can help healthcare organizations optimize their operations and reduce costs.
- Clinical trial data: Clinical trial data refers to data collected during clinical trials of new drugs, treatments, or medical devices. Clinical trial data can be used to evaluate the effectiveness and safety of new treatments, and it can inform clinical practice guidelines and treatment decisions.
- Public health data: Public health data refers to data on population health, including data on disease prevalence, environmental factors, and social determinants of health. Public health data can be used to identify health disparities, track disease outbreaks, and inform public health policies and interventions.
- Patient-generated data: Patient-generated data refers to data collected directly from patients, including data from wearables, mobile apps, and patient-reported outcomes. Patient-generated data can be used to monitor patients remotely. Identify trends in patient behavior and health status, and personalize treatment plans.
Overall, healthcare data sources are critical to improving patient outcomes and optimizing healthcare operations. By leveraging these data sources and using data analytics and machine learning techniques. Healthcare organizations can make more informed decisions about patient care and improve healthcare delivery.
Predictive Analytics in Healthcare
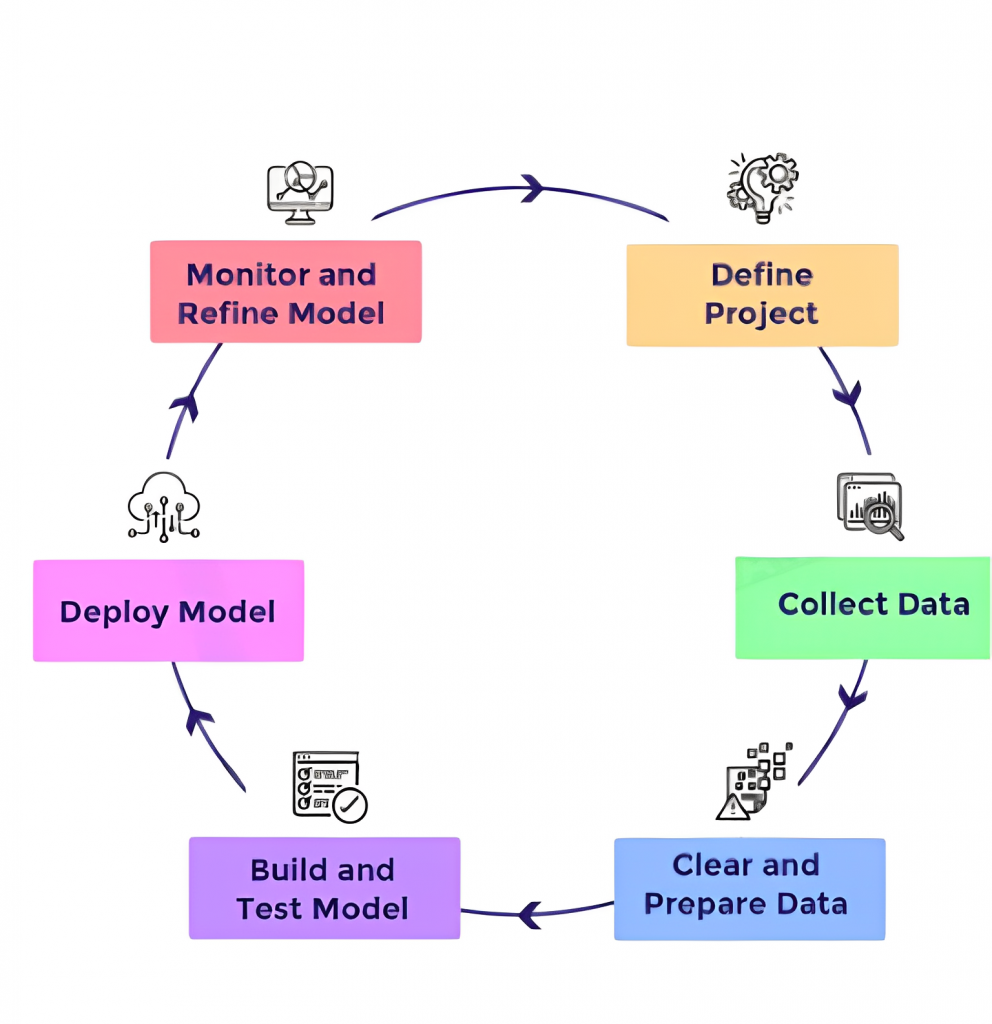
Predictive analytics in healthcare is the use of statistical algorithms and machine learning techniques to identify patterns and predict future outcomes based on historical data. By analyzing large amounts of patient data, predictive analytics can help healthcare organizations and clinicians make more informed decisions about patient care, leading to better outcomes and improved efficiency.
One example of the use of predictive analytics in healthcare is the prediction of readmission rates. By analyzing patient data, including demographics, medical history, and lab results, predictive analytics can identify patients who are at high risk of readmission. This allows clinicians to provide targeted interventions, such as medication management or care coordination, to reduce the likelihood of readmission and improve patient outcomes.
However, there are several difficulties in using predictive analytics in the healthcare industry. Ensuring the moral and secure use of patient data is a challenge. Another problem is the potential for bias in the data used to create predictive models, which might lead to inaccurate predictions and bad treatment decisions.
Another example of the use of predictive analytics in healthcare is in the development of personalized treatment plans. By analyzing patient data, including genetics, medical history, and lifestyle factors, predictive analytics can help clinicians develop more personalized treatment plans for individual patients. This can lead to improved treatment outcomes and reduced healthcare costs.
However, there are challenges associated with the use of predictive analytics in healthcare. One challenge is ensuring the ethical and safe use of patient data. However, healthcare professionals need to ensure that they handle patient data securely and ethically and that they receive adequate training to apply data analytics in their practices.
Overall, the use of predictive analytics in healthcare has the potential to improve patient outcomes and transform the healthcare industry. However, it is important to address these challenges and develop ethical frameworks for the use of predictive analytics in healthcare.
Improving Patient Outcomes with Data Analytics
In addition to reducing readmission rates, data analytics has the potential to improve patient outcomes in many other ways. For example, data analytics can help clinicians identify patients who are at risk of developing chronic conditions such as diabetes, heart disease, and hypertension. By monitoring these patients closely and providing them with personalized interventions, such as lifestyle changes and medication management, clinicians can help prevent the onset of these conditions or manage them more effectively if they do develop.
Data analytics can also help improve clinical decision-making by providing clinicians with more comprehensive and accurate patient information. For example, electronic health records (EHRs) can provide clinicians with access to a patient’s medical history, lab results, and imaging data, all in one place. By analyzing this data and identifying patterns and trends, clinicians can make more informed decisions about a patient’s care, leading to better outcomes.
Another potential use of data analytics in healthcare is in the development of precision medicine. Precision medicine involves tailoring medical treatment to the individual characteristics of each patient, including their genetics, lifestyle, and environment. By analyzing large amounts of patient data and identifying patterns, data analytics can help clinicians develop more personalized treatment plans for individual patients.
Finally, data analytics may assist healthcare firms in streamlining their processes and lowering expenses. Hospitals can use data analytics to detect wasteful resources and workflows, leading to reduced spending and improved patient flow.
Overall, data analytics has the potential to revolutionize the healthcare sector and greatly enhance patient outcomes. However, healthcare professionals need to ensure that they handle patient data securely and ethically and that they receive adequate training to apply data analytics in their practices.
conclusion
Datafication has the potential to revolutionize patient care in healthcare by providing clinicians with valuable insights into patient health, enabling more accurate diagnoses, and improving treatment outcomes. Data analytics, including machine learning and predictive analytics, can help identify patterns and trends in patient health data that may not be apparent to human clinicians, leading to more accurate diagnoses and better treatment plans.
Healthcare is becoming increasingly data-driven, but there are drawbacks as well, such as worries about data security and privacy, potential bias in the data used to train predictive models, and high implementation costs for EHRs. Despite these difficulties, datafication has a huge potential to enhance patient outcomes and change the healthcare sector.
Moving forward, it will be important to continue to address these challenges and develop ethical frameworks for the use of data analytics in healthcare. By doing so, we can harness the power of datafication to improve patient outcomes, reduce costs, and transform the healthcare industry.